Hay 18 vacantes para Sql
Hace más de 30 días.
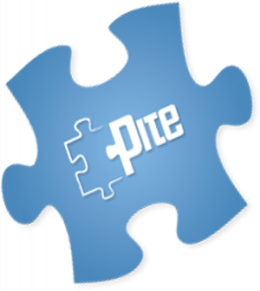
Desarrollador BackEnd SQL Middle - Remoto en ´PITE
$1125 USD
Únete a nuestro equipo para desarrollar y mantener aplicaciones web, optimizar código, gestionar bases de datos y guiar a otros con conocimientos básicos de IA.
Hace más de 30 días.
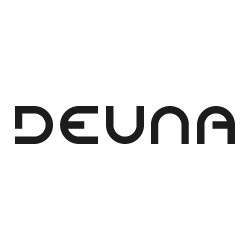
Data Engineer SQL Senior - Remoto en DEUNA
Sueldo oculto
Únete a DEUNA como Sr Data Engineer y transforma el e-commerce en LATAM. Impulsa ETL escalables y modelos de datos con tecnologías líderes. ¡Postula ahora!
Hace más de 30 días.
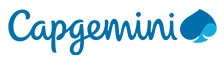
ADF/ETL Senior SQL - Remoto en Capgemini Mx
Sueldo oculto
Únete a nuestro equipo como experto en MS Azure y MSBI. Desarrolla y automatiza aplicaciones complejas en un entorno ágil. ¡Explora más sobre esta oportunidad!
Hace más de 30 días.
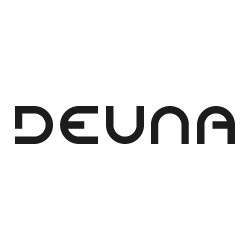
Technical Project Manager SQL Middle - Remoto en DEUNA
$5250 USD
Únete a DEUNA, una startup SaaS en expansión con $30M en financiamiento, y lidera proyectos técnicos en e-commerce en LATAM. ¡Buscamos tu talento!
Hace más de 30 días.
Desarrollador WinDev con experiencia en SQL - Remoto en Ambit
$1375 USD
¡ÚNETE A NUESTRO EQUIPO! Buscamos Desarrollador experto en Windev para proyectos dinámicos. Ofrecemos trabajo remoto, crecimiento y capacitación. ¡Envía tu CV hoy!
Hace más de 30 días.
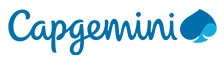
Especialista en Soporte de Infraestructura Informática (SQL) - Senior - Remoto en Capgemini Mx
Sueldo oculto
Únete a nuestro equipo como IDQ Developer: supervisa trabajos de producción, gestiona servicios IDQ/MDM y participa en actividades de migración y parcheo. ¡Aplica ahora!
Hace más de 30 días.
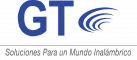
Fullstack Developer Senior - Remoto en Gta Telecom De México
Sueldo oculto
Únete a nuestro equipo para desarrollar aplicaciones innovadoras con .NET Core y SQL Server. Buscamos expertos con 8+ años de experiencia en software y habilidades en JavaScript y Agile.
Hace más de 30 días.
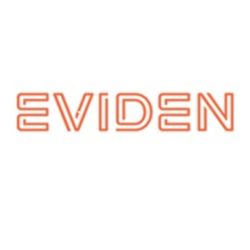
QA Engineer Senior - Hybrid (Manual and Automation) - Remoto en Eviden
$2375 USD
Únete como Hybrid QA Engineer: 4+ años en QA, SQL, JavaScript y pruebas móviles. Experiencia en APIs REST y automatización. Ubicación: México. Inglés avanzado requerido.
Hace más de 30 días.
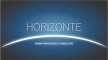
Desarrollador Databricks - Remoto en Capital Empresarial Horizonte
$3250 USD
¡Únete a Capital Empresarial Horizonte como Desarrollador Databricks! Trabajo remoto, salario competitivo, beneficios atractivos y oportunidades de crecimiento. ¡Aplica ahora!
Hace 3 días.
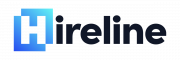
Desarrollador Fullstack Sr con NodeJs - Remoto en HIRELINE
$4250 USD
Únete como Ingeniero de Software Senior en un entorno remoto, usando Node.js, Angular y AWS. Diseña y escala sistemas innovadores con autonomía y colaboración efectiva.
Hace más de 30 días.
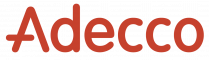
Dynamics Support Great Plains ERP Platform Senior - Remoto en Adecco
$2750 USD
Join us as a Dynamics Support Specialist for Great Plains ERP! Enjoy a 100% remote role in Mexico with a competitive salary and excellent benefits. Apply via WhatsApp!
Hace más de 30 días.
Consultor ETL ODI SR. - Remoto
Sueldo oculto
Únete a Derevo como Consultor ETL ODI y libera el poder de los datos. Ofrecemos trabajo remoto, desarrollo interdisciplinario y proyectos internacionales. ¡Postúlate ahora!